Artificial intelligence has been in the center of attention for quite a while now. But, as it turned out, people talk more about it then they work on it: MMC, a venture capital firm in London, dug into the works of 2,830 AI startups and what they found was that 40% of them didn’t actually have the characteristics of AI.
Setting fake and real AI startups apart is not an easy task for big companies either. As the story goes, Mcdonalds acquired an AI firm called Dynamic Yield for $300 million in the hope of advancing its customer service through machine learning. It turned out later that the company was not working on real AI at all, according to the company’s former member.
What causes so much misunderstanding?
Based on the capabilities of Artificial Intelligence, it’s no wonder why it got so much attention. Startups, investors, and various companies all got into this space without fully understanding what it actually was. And, that’s where the misunderstanding started. Behind the beautiful slogans here are standard scripts hiding most of the time. We have seen such bubbles in the past, too. The same happened with Blockchain and the same was the .com bubble. The investors didn’t even know what the proper use of domain names was, so they bought them at unrealistically high prices. The same is happening now too.
Artificial Intelligence vs. Scripts
Even though the difference between an AI system and a standard script is huge, the border between them is still quite blurry. Especially for those who have no technological background.
Today one can write very complex programs using a standard script. The difference between that and AI is that the latter can analyze the data acquired in real-time and evolve accordingly. This is enabled through machine learning and deep learning mechanisms. For example, Google’s Artificial Intelligence offers users recommendations based on their individual search history. Before, it simply offered us the most popular texts.
Another good example is online chatbots. If the system can’t go over pre-determined answers, then it’s a script-based chatbot. In comparison, an AI chatbot can remember the information acquired from each interaction, analyze the results, adapt accordingly, and use the information again in the future. This means that it can recognize a returning client, remember what issues they had before, and communicate with them more efficiently.
But, as mentioned before, today it is very easy to write complex scripts which makes it harder to tell the difference. There are a couple of details, though, which one should pay attention to to better evaluate an AI firm.
5 main questions for an AI company
-
Who is the team made up of?
Just as in the case of any other type of company, the team is a crucial element for success. Designing an AI program requires a lot of skill and experience - and not in only one direction.
Strong AI team has to have three types of specialists: Programmers, who comfortably work on Python, C++, Java, Lisp, or Prolog, data engineers, and field experts. If the team consists of one or two types of specialists, then they’ll need to find new members for the development of the project.
-
Questions about data
For the system to be able to effectively learn from past experiences, it needs a lot of data sets. The internet and social media have simplified the process a lot, but this doesn’t mean that it doesn’t require a lot of time and effort. Because data is an important part of AI development, there are a couple of questions to be asked here:
2.1) How much data did you use to train your AI and where did you get them from?
Everything starts with gathering the data. If the startup can’t access the necessary information, then this will be a huge bump on the road. Ask them to tell you where they got the data from and how they settled on the necessary amount. Most of the time, data scientists feed data to the system repeatedly until they reach the desirable outcome (but, there are other strategies too).
There is no fixed amount of data required to train AI. This depends on task difficulty and other external factors.
To have a better idea, in this graph you’ll see the amount of data that was used for different types of AI companies:
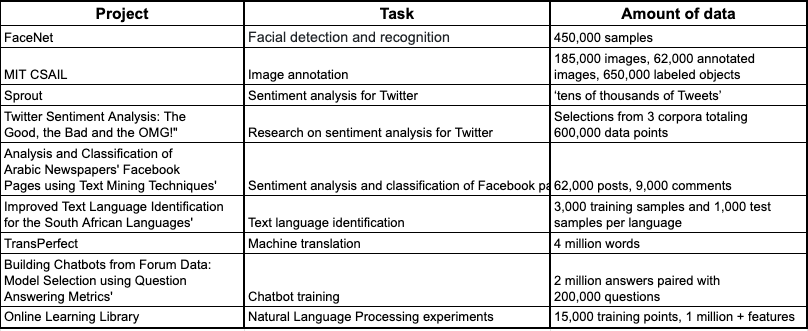
Source: lionbridge.ai
2.2) How qualitative is your data?
When talking about the data, the quality turns out to be just as important as the quantity. To make proper predictions the system should be able to clearly understand the context of the data. For this they need to be well labeled, and that can be seen as the main task of data engineers. One could even say that quality is more important than the quantity. 100 labeled data will be more useful for the system than a 500 unlabeled.
2.3) How often should the system be fed with new data and how are you planning to do this technically?
It is true that Artificial Intelligence mostly works independently from humans but it still needs some maintenance periodically. If a startup presents its AI as a superhero who never needs human’s help, then there’s a big chance they don’t really understand how the system actually works.
For more detailed information about data use in AI refer to this article.
-
What problems did you stumble upon during the development and implementation processes?
Every new system, especially the kind that represents a completely new technology, stumbles upon many challenges along the way. Those who supervise the process know this well. Ask the project initiators to explain in detail what did not turn out the way they expected it to and what measures they took to work around the issue.
-
How much time and financial resources went into the development and who has examined it?
If the startup is new, then the system is probably incomplete and in the early stage of development. In an ideal situation, the company has to be a couple of years old with a lot of funds backing it. It’s a big bonus if it’s already tested in the real business world.
Here it is important to consider on which stage of the project development would you like to invest. A startup whose early results look promising might be worth attention. But, if the team members aren’t that experienced and if the AI system hasn’t left the lab yet, then you have to be ready to invest a lot of effort and money for success.
-
Has the system been used in the real business world and if so, what results has it provided?
This question will not help you that much in determining the validity of the project but it will help you better understand how developed it is and how big its chance to success are.
Initially, an AI system is not as efficient in our chaotic reality as it is in the lab where external factors are more controlled. If the startup already has an experience in this then they will tell you exactly what problems they stumbled upon during real world testing and what measures they took to solve them.
As it is often mentioned, only around 20% of AI startups hardly ever leave the lab. This is even less than the amount of real AI companies.
Be careful with AI companies!
20 August 2020Artificial intelligence has been in the center of attention for quite a while now. But, as it turned out, people talk more about it then they work on it: MMC, a venture capital firm in London, dug into the works of 2,830 AI startups and what they found was that 40% of them didn’t actually have the characteristics of AI.
Setting fake and real AI startups apart is not an easy task for big companies either. As the story goes, Mcdonalds acquired an AI firm called Dynamic Yield for $300 million in the hope of advancing its customer service through machine learning. It turned out later that the company was not working on real AI at all, according to the company’s former member.
What causes so much misunderstanding?
Based on the capabilities of Artificial Intelligence, it’s no wonder why it got so much attention. Startups, investors, and various companies all got into this space without fully understanding what it actually was. And, that’s where the misunderstanding started. Behind the beautiful slogans here are standard scripts hiding most of the time. We have seen such bubbles in the past, too. The same happened with Blockchain and the same was the .com bubble. The investors didn’t even know what the proper use of domain names was, so they bought them at unrealistically high prices. The same is happening now too.
Artificial Intelligence vs. Scripts
Even though the difference between an AI system and a standard script is huge, the border between them is still quite blurry. Especially for those who have no technological background.
Today one can write very complex programs using a standard script. The difference between that and AI is that the latter can analyze the data acquired in real-time and evolve accordingly. This is enabled through machine learning and deep learning mechanisms. For example, Google’s Artificial Intelligence offers users recommendations based on their individual search history. Before, it simply offered us the most popular texts.
Another good example is online chatbots. If the system can’t go over pre-determined answers, then it’s a script-based chatbot. In comparison, an AI chatbot can remember the information acquired from each interaction, analyze the results, adapt accordingly, and use the information again in the future. This means that it can recognize a returning client, remember what issues they had before, and communicate with them more efficiently.
But, as mentioned before, today it is very easy to write complex scripts which makes it harder to tell the difference. There are a couple of details, though, which one should pay attention to to better evaluate an AI firm.
5 main questions for an AI company
Who is the team made up of?
Just as in the case of any other type of company, the team is a crucial element for success. Designing an AI program requires a lot of skill and experience - and not in only one direction.
Strong AI team has to have three types of specialists: Programmers, who comfortably work on Python, C++, Java, Lisp, or Prolog, data engineers, and field experts. If the team consists of one or two types of specialists, then they’ll need to find new members for the development of the project.
Questions about data
For the system to be able to effectively learn from past experiences, it needs a lot of data sets. The internet and social media have simplified the process a lot, but this doesn’t mean that it doesn’t require a lot of time and effort. Because data is an important part of AI development, there are a couple of questions to be asked here:
2.1) How much data did you use to train your AI and where did you get them from?
Everything starts with gathering the data. If the startup can’t access the necessary information, then this will be a huge bump on the road. Ask them to tell you where they got the data from and how they settled on the necessary amount. Most of the time, data scientists feed data to the system repeatedly until they reach the desirable outcome (but, there are other strategies too).
There is no fixed amount of data required to train AI. This depends on task difficulty and other external factors.
To have a better idea, in this graph you’ll see the amount of data that was used for different types of AI companies:
Source: lionbridge.ai
2.2) How qualitative is your data?
When talking about the data, the quality turns out to be just as important as the quantity. To make proper predictions the system should be able to clearly understand the context of the data. For this they need to be well labeled, and that can be seen as the main task of data engineers. One could even say that quality is more important than the quantity. 100 labeled data will be more useful for the system than a 500 unlabeled.
2.3) How often should the system be fed with new data and how are you planning to do this technically?
It is true that Artificial Intelligence mostly works independently from humans but it still needs some maintenance periodically. If a startup presents its AI as a superhero who never needs human’s help, then there’s a big chance they don’t really understand how the system actually works.
For more detailed information about data use in AI refer to this article.
What problems did you stumble upon during the development and implementation processes?
Every new system, especially the kind that represents a completely new technology, stumbles upon many challenges along the way. Those who supervise the process know this well. Ask the project initiators to explain in detail what did not turn out the way they expected it to and what measures they took to work around the issue.
How much time and financial resources went into the development and who has examined it?
If the startup is new, then the system is probably incomplete and in the early stage of development. In an ideal situation, the company has to be a couple of years old with a lot of funds backing it. It’s a big bonus if it’s already tested in the real business world.
Here it is important to consider on which stage of the project development would you like to invest. A startup whose early results look promising might be worth attention. But, if the team members aren’t that experienced and if the AI system hasn’t left the lab yet, then you have to be ready to invest a lot of effort and money for success.
Has the system been used in the real business world and if so, what results has it provided?
This question will not help you that much in determining the validity of the project but it will help you better understand how developed it is and how big its chance to success are.
Initially, an AI system is not as efficient in our chaotic reality as it is in the lab where external factors are more controlled. If the startup already has an experience in this then they will tell you exactly what problems they stumbled upon during real world testing and what measures they took to solve them.
As it is often mentioned, only around 20% of AI startups hardly ever leave the lab. This is even less than the amount of real AI companies.